Vibe-Tuning Llama on SimpleQA and Severance
Automating an agentic pipeline for synthetic data generation — no labels, just agents
✦ TL;DR
I fine-tuned Meta Llama (3.1-8B) on Severance using an agentic pipeline, generating high-quality synthetic data with zero manual labels. The results? My tuned Llama model answered 7 out of 14 questions correctly. The base model got 0. Here's how the models stacked up in evaluation (GPT-4o, base Llama, tuned Llama). Not surprisingly, GPT-4o outperformed the others (9 out of 14 correct). It's the largest model of the bunch and has likely seen these examples in training.
But here's the catch: in an enterprise setting, with your private data, GPT-4o would likely underperform because it hasn't been trained on your domain. Finetuning a smaller model on your proprietary data doesn't just boost accuracy, it gives you a domain-specific model with lower latency and cost.
The key to finetuning? Start small, carefully scope your data, let agents do the heavy lifting, and iterate like your accuracy depends on it (because it does).
Check out our upcoming webinar on generating high-quality synthetic data on April 30, 👉 register now 👈.

Introduction
Severance is one of my favorite shows. It’s about grief and loss, memory and love, identity and purpose — and the meaning of work and life. On the surface, it’s a workplace thriller. But underneath, it’s a meditation on what makes us us — and what happens when memory gets severed from identity.
That made it the perfect subject for my first serious finetuning project.
I’m a designer with a background in psychology and HCI, not an ML engineer. However, at Lamini, I design experiences for developers to adapt LLMs to their specific use cases and data — and I realized I couldn’t design for our users unless I became one myself. So I gave myself a challenge: use our agentic pipeline and Memory Tuning to teach Llama to answer factual questions about Severance.
This post is a reflection on this journey — automating a factual QA pipeline, finetuning with synthetic data, and learning what it actually takes to teach an LLM, one agent at a time.
Why teaching LLMs facts is hard
LLMs don’t “know” facts. They predict the next word, meaning they’re “designed” to hallucinate, especially when working across multiple documents or sources, and even conflicting or ambiguous ones.
The nature of Fact:
- It’s subjective, a single sentence can contain multiple micro-facts.
- Hard to evaluate factuality, unless you’re an expert in your data. LLM-as-a-judge? What if that LLM doesn't know much about what you’re trying to evaluate?
The nature of LLMs:
- LLMs learn from patterns, not truth.
- Non-deterministic. Because it’s all about probability, even a slight wording change can cause a completely different output.
Why finetuning?
Again, prompting can’t work, especially, if the model hasn’t seen the facts before, it will just hallucinate based on what it has been taught.
RAG might get you to 80% accuracy, but if you're aiming for 90%+, it starts to break down:
- It can’t resolve subtle conflicts between documents.
- It struggles with consistent answers across edge cases — still non-deterministic
- Inference can be slow, especially when retrieving across many documents.
Finetuning solves that by teaching the model to memorize the facts. It doesn't just reference knowledge temporarily — it actually learns it (by embedding extra weights on top of the model). That’s how you move from “it kinda knows” to “this model knows what it’s talking about.”
Finetuning is hard though, especially for fact memorization. Here, we use Lamini’s Memory Tuning, a finetuning technique that embeds new knowledge into the LLM and makes retrieval fast and efficient. Memory Tuning uses two technologies — LoRAs (Low Rank Adaption) and MoE (Mixture of Experts) and enables you to turn any open source LLM into a Mixture of Memory Experts. Learn more about Memory Tuning.
Lessons from vibe-tuning Llama on Severance
1. Start small. No, smaller.
OpenAI’s SimpleQA is specifically built to test factual recall — the kind of basic knowledge even GPT-4o can still struggle with.

SimpleQA has 4.3k records, each with a question, answer, metadata, and multiple URLs. It covers a wide range of topics and answer types. It spans a wide range of topics and answer types, so I figured it would be a solid starting point for building my evaluation dataset—the ground truth I’d use to assess model performance.
But after a closer look, I realized the data wasn’t as clean as I’d hoped. There were broken links, duplicate URLs, and even conflicting sources within the same record.
And 4.3k records? Definitely too much to start with. So I narrowed it down: first by topic — TV shows. But even that turned out to be too broad. My early experiments showed that because facts are often subjective, and LLMs are inherently non-deterministic, the model would produce inconsistent results.
So I scoped even further: TV shows → Severance → QA pairs where the answer type is “Person” (i.e., character names) which has 14 QAs that I later used to evaluate my tuned models
That was the turning point. With a smaller scope, I could control the data quality, understand the outputs better, and evaluate results much faster — all essential for iteration.
2. Find the right teaching materials
Once I started reviewing generated QA pairs and also the original content (URLs), I noticed a few issues:
- There were many duplicated QAs
- Similar content, different URLs were used, for example:
a. https://severance-tv.fandom.com/wiki/Mark_Scout
b. https://severance.wiki/mark_scout
These might have created redundancy, inconsistency, and sometimes conflicting context.
So I paused, took a step back, and I asked myself, if I want to teach LLama about Severance, what would be the most useful materials? Instead of using the URLs provided in the SimpleQA eval, I built my own URL list, primarily from https://severance-tv.fandom.com/wiki/Severance_Wiki:
- The show’s main overview page
- Character pages
- Episode pages
I also experimented with character only pages. You want to give the model enough relevant information, but not overwhelm it, because if there are multiple sources for similar topics, the model can get confused.
3. Own your pipeline — one agent at a time
At first, I borrowed a pipeline my coworker had built for a customer. It included multiple generators and validators and was designed for a similar factual QA use case. I ran it as-is… and quickly hit a wall.
With so many outputs from different generators, it was nearly impossible to iterate quickly or know what was going wrong.
At Lamini, we’re building default pipelines for different use cases such as Text-to-SQL and factual QA — you can just connect your source data to the pipeline, run it, and get a good first run accuracy. What I learned was that each use case is unique so you still need to adjust each agent accordingly.
So I hit reset.
I stripped it down to just one QuestionAnswerGenerator. I ran the pipeline, manually reviewed the output (vibe check!), and started refining the prompt. That made everything faster and clearer. The feedback loop got tighter, and I could focus on tuning one agent at a time.
Agentic pipelines are fragile — if one agent fails, especially early in the chain, the whole thing breaks. So, again, start small, one agent at a time, add more if needed, and make sure that each agent’s job description is clear and they are performing their job correctly.
4. Experiments and iteration
This part was the most challenging — and the most fun. For a week straight, I lived inside the loop: Generate → Error Analysis → Prompting Engineering → Repeat.
I started with vibe checks, but eventually moved to more serious error analysis — figuring out why the model got the wrong answers. Did my pipeline generate relevant QAs? Was there conflicting information? Was my tuning working?
I tweaked prompts and tuned hyperparameters, watched hallucination rates go down, and accuracy go up. Eventually, my best tuned model got 7 out of 14 eval questions right.
While waiting on tuning jobs, I also tested our Memory RAG. It was faster and surprisingly effective — a good reminder that you don’t always need finetuning to iterate. But when you do want max accuracy, finetuning still wins.
One big lesson: ML is not software engineering. In software, commits are forever. In ML, most experiments get thrown away. And that’s the point.
5. Tweak everything: Prompts, agents, models, chunking
1. Prompts (aka, prompt engineering)
Even small tweaks in prompt phrasing can make a huge difference. I spent a lot of time refining instructions, breaking down steps, adding examples. One word can make or break the output — especially for models that like to go off-script.
You may want to experiment with:
- Few shot prompting: basically, add a few examples; especially, if the model doesn't handle edge cases well, you may want to add that into the prompt.
- Chain of thought: ask the model to think step by step, or provide specific step-by-step instructions, or even add a few examples.
Here is the final prompt for my QuestionAnswerGenerator. I already have many rules, but guess what, LLMs are like a naughty kid, sometimes it listens, sometimes, it completely ignores your rules, so continuing to add more specific rules won't help.
You can see that my prompt is huge. My next step is to think about breaking it down to smaller generators. But it was just so fast to iterate with one generator.
2. Generators and validators
You can plug in different generators to create QA pairs, and different validators to clean or filter them. Prompting strategies matter for each one — whether it’s a generator or a validator. For example, in our demo text-to-SQL pipeline, we used a combination of agents like:
- SchemaToSQLGenerator
- SQLDebuggerGenerator
- PatternQuestionGenerator
- VariationQuestionGenerator
- QuestionDecomposerGenerator
- SQLValidator
Check it out here: Lamini Text-to-SQL Examples
Generating high-quality QAs is important, meanwhile, making sure your dataset has a good balance among all the concepts and facts, if a model sees one concept much more than another one, it may likely produce an answer using the former concept — well, it’s all about probability and prediction right?
That said: start small. Seriously. Use as few agents as possible — maybe even just one generator to begin with. You’ll quickly figure out whether you need to break it down into smaller components or add more validators later. Simpler pipelines are easier to debug, faster to iterate on, and much easier to vibe-check.
Llama or Deepseek?
I used a single model for generation, validation, and tuning. But that’s something I want to change — different models might be better for different pipeline stages. There’s real potential in specializing models per task.
I also have a next-step idea: train a model that’s really good at prompt engineering itself — basically, a prompt optimizer. That way, I don’t have to keep manually adjusting and error-checking with ChatGPT. You can literally fine-tune a model to act as an agent for any task.
Chunking strategy
How does the generation work? The model reads each given chunk and generates QAs based on the prompt, so of course how you chunk the content matters. You can experiment with different chunk sizes, SentenceChunker or TokenChunker, or add more metadata to the chunk to make it more informative. We’re building some smart chunking so you don't have to worry about this.
6. The ML bit: Hyperparameters tuning
This was the most "ML" part of the process — and where I learned the hard way.
You can have perfect data and still end up with a bad model if your tuning config is off. That’s the magic (and pain) of hyperparameters.
The goal?
Let your model overfit on your training data first — then see if it can generalize.
One example from my own tests:
From training set: Who became the CEO of Lumon in 2013? → James Eagan ✅
Non training set: Who is the CEO of Lumon in 2013? → Kier Eagan ❌
Same question, slightly reworded — totally different result. That’s an overfit model that can’t generalize.
Variables worth experimenting with:
- Learning rate
- Max steps / epochs
- Base model (size and architecture)
The more I tweaked, the better I understood how the model actually learns — and where it breaks.
7. LLMs: Smart and stupid
LLMs’ ability to generalize is what makes them both magical and maddening.
Same prompt, different phrasing → different answer.
With or without a PromptTemplate → totally different behavior.
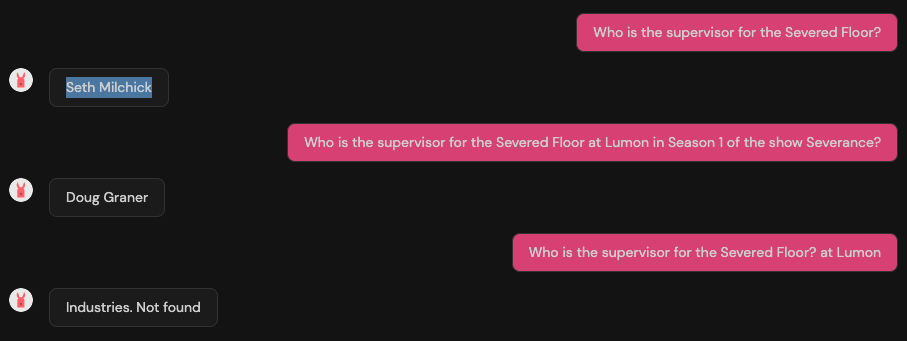

My favorite one from my generated data,
Question: Who introduces themselves as Nina at the bar?
Answer: Nina.
Yes, my name is Nina. Not wrong. Just... hilarious…
8. Next steps
How do you teach a model more?
Think back to how you learned as a kid: step by step, concept by concept, with examples, practice, and feedback. Teaching LLMs isn’t so different.
Right now, I’m only finetuning Llama on Severance character questions — where the answer is always a person. But there’s more ground to cover: episodes, dates, relationships, numbers. Now that I have a solid agent for “Person,” my plan is to build similar generators for other answer types — starting with prompt tweaks and maybe adding validators where needed.
I’m also still learning the deeper ML side — especially hyperparameter tuning and figuring out how much is “good enough.” But the bigger question is: how do we make this easy for everyone, not just ML experts?
And yes, I’ve felt the pain: tracking experiments, managing config chaos, doing deep error analysis manually. It’s clear we need better tools — and we’re working on it.
Final thoughts
So, what did I learn?
- Start small — then go smaller
- The right teaching materials matter
- Own your pipeline
- One agent at a time
- Experiment like your model depends on it (because it does)
- Embrace the chaos. Laugh at hallucinations.
I now fully understand the pain our users feel — and I also see how far we’ve come. A few years ago, finetuning an LLM like this would've required a research team. Now? A designer can do it in a few weeks.
Of course, I’m still at the start. And factual QA? Way harder than Text-to-SQL (which I also tried!). But if a designer can teach Llama 3 to beat ChatGPT on even a handful of Severance questions — imagine what developers, and eventually anyone, will be able to do next.
RESOURCES
- SimpleQA: https://openai.com/index/introducing-simpleqa/
- Agentic Pipeline/Memory Experiments: https://docs.lamini.ai/memory_experiments/
- Eval Guide: https://docs.lamini.ai/memory_experiments/evaluation/
- Prompt Engineering Guide: https://www.kaggle.com/whitepaper-prompt-engineering
ACKNOWLEGEMENT
Last but not least, huge credit to all my co-workers who helped me on my journey to becoming a model trainer 😄 🙌